IPB Professor: IPB Develops Artificial Intelligence to Predict Rain
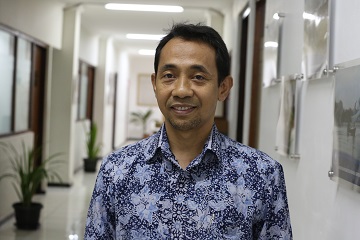
The search for a solution to a problem now begins to shift from using mathematical formulas to using computerized computational. Mathematical formulas are no longer used, especially when facing something that is not clear, problems that are too complex or uncertainty during the system process.
Computational has emerged since a long time ago, while the storage device was not existed yet. In early 1900, a vacuum tube with the size of a human thumb was discovered. After 1970, a smaller transistor was discovered. Now, the size of one transistor is only seven nanometers.
"The influenza virus is 100 nanometers in size, so the transistor is 1/15 the size of the influenza virus. Hopefully, a transistor’s size can decrease to be one atom, so quantum mechanics can be used. That is what is meant by quantum computational. This means that the size of transistor will be even smaller, 415 times smaller than the influenza virus. Well, I need to say this because with the emergence of computational power, this changes the paradigm in problem solving," said Prof. Dr. Agus Buono, Professor of the Faculty of Mathematics and Natural Sciences, Bogor Agricultural University (FMIPA-IPB) during a Press Conference Pre Scientific Oration at IPB Baranangsiang Campus, Bogor, (2/8).
Therefore, there are several new sciences such as Artificial Intelligence (AI). Research conducted by Prof. Agus Buono focuses on computational intelligence (Center for Artificial Intelligence / CAI). It is finding a solution to a problem by imitating the way living things solve their problems.
"For example, how ants can find the shortest path. Or birds in the cloud can see the food source. Or how our bodies can recognize foreign agents and make antibodies mold immediately. Those are natural intelligences that computer science experts imitate to make algorithms," he explained.
According to him, currently, artificial intelligence has developed into five ways: neuro model computational (imitating brain intelligence), evolutionary programic (how to choose one solution from a variety of solutions), colony intelligence (imitating the behavior of a group of animals to get optimum things), and fuzzy model (how to make a soft algorithm). Nowadays, artificial intelligence is used to quantify a trait, for example the expertise of a doctor is modeled in fuzzy model where algorithms can recognize attacks in a system.
In agricultural context, Prof. Agus Buono does computation to predict rain. Prof. Agus inserted all variables related to rain. The variables are the value of daily and monthly rain or when it starts to rain, the length of the rainy season or how much it rains or how it distributes four to five consecutive rainy days or extreme rain and so on.
"Well, for rain prediction, there are usually several types of data included, such as satellite data and models and observation data. Each of these data has its weaknesses. Satellite data is real time and spacial but sometimes not in accordance with real conditions in the field. While the observation data is in accordance with the conditions in the field, the dataitself is incomplete. So I put these two data together. Later, this rain prediction will be useful for agriculture, aviation and others," he said.
Based on the results of his research in Pacitan, with the modification of CNN (Computational Neuron Network) to be suitable for temporal predictors (the team called it TD CNN), the team managed to avoid false rain (which is usually followed by drought).
"There was less than 50 millimeters of rain after the dry season and farmers suspected that it was a sign for the beginning of rainy season so they started to plant rice. But it turns out that the rain is false rain or no rain in the next three days. So there will be drought at the beginning of the planting season because of false rain. Our TD CNN results are better than predictions with other methods," he said.
From these findings, Prof. Agus concluded that computational intelligence can be part of artificial intelligence to realize an agriculture that is predictable, anticipatable, controllable, personalizeable, real time and precise in the production, processing and distribution phases, for efficiency, effectiveness and sustainability. (RYF)